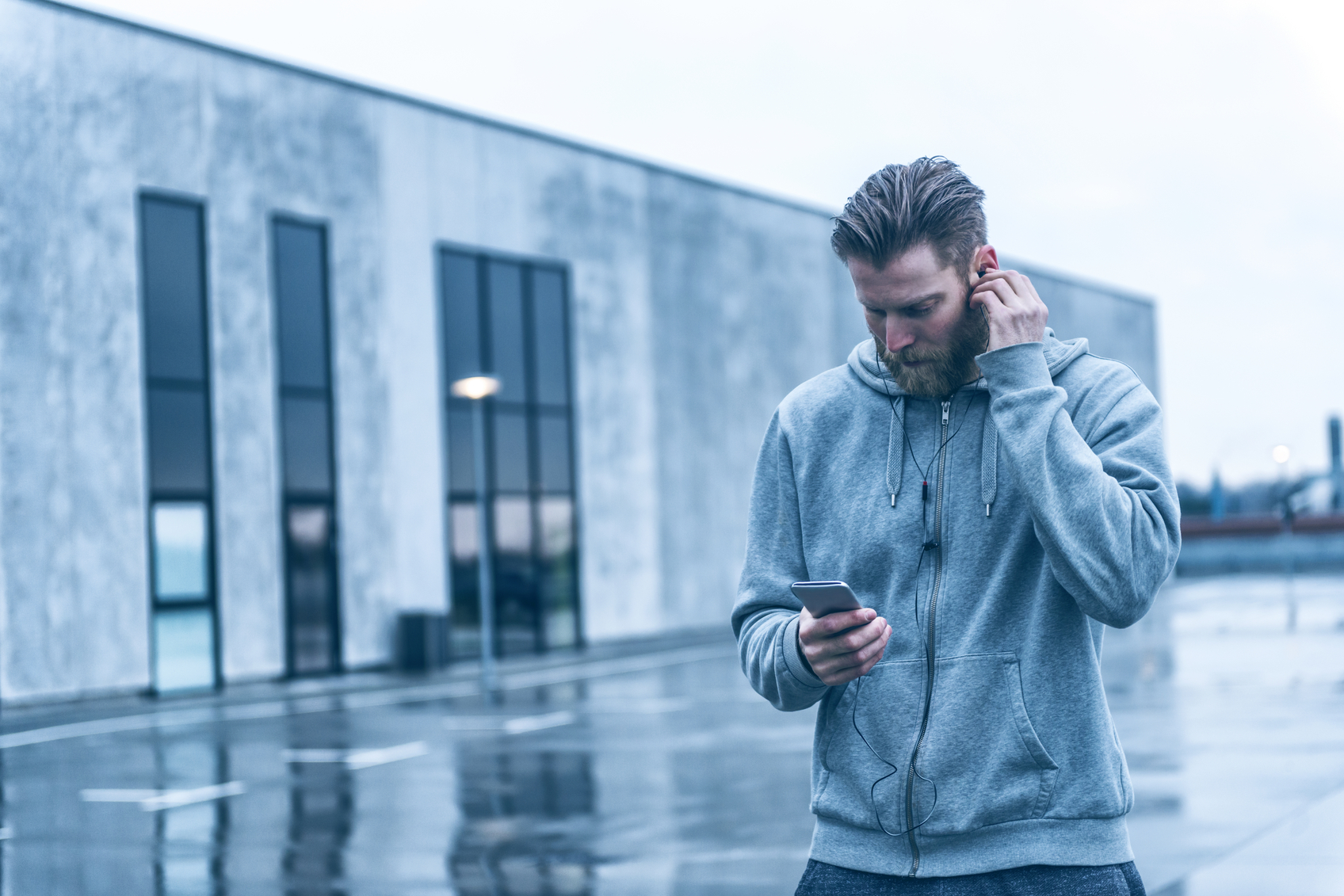
Improving both patient outcomes and operational efficiency is perhaps the ultimate one-two punch for most healthcare providers, and it’s becoming increasingly apparent that AI has a critical role to play.
In a recent interview with HealthcareITNews, however, Phoebe Yang, general manager for non-profit healthcare at Amazon Web Services, noted that beyond the technological specifics, it’s interoperability of data that is the real key.
“Interoperability among medical information systems is foundational – or should be – because without it, physicians don’t have ready access to their patients’ complete medical histories,” Yang explained. “Failing to bring all information together undermines clinical teams’ diagnoses and treatment plans. It opens the door to poor outcomes and readmissions that harm both the patient and the overall cost of running the system.”
Moreover, Yang argued, the goal shouldn’t be to reduce medical errors. Indeed, she noted, “(h)ealthcare is more clinically effective and cost-effective when it prevents problems instead of reacting to them. Machine learning fueled by comprehensive data can help physicians predict health issues across populations and in individual patients. A timely view of the whole patient can drive breakthroughs in personalized medicine and close gaps in care, including disparities for the most vulnerable people. Data and AI/machine learning are the keys to making that happen.”
Not surprisingly, Yang also pointed to the importance of the cloud, due primarily to its ability to support the massive volumes of data AI relies on.
For example, she said, in cancer care “(r)educing the cost and complexity associated with managing large pathology-image volumes is making critical data readily available to researchers and clinicians, all with the goal of accelerating research and improving diagnoses.”
According to Yang, the ultimate benefit of the array of new technologies is their capacity to give providers a comprehensive view of patients. In short, data liquidity “can help practitioners combine an individual patient’s own data, at new levels of completeness and detail, with anonymized large-population patient data, including genomics. AI/machine learning-based learning models can use that large, detailed view to predict specific, individual health threats with unprecedented accuracy.”
As to how this level of personalized medicine might play out on the ground, Yang noted, for example, the “information that an algorithm uses to predict a heart attack may originate in a smartphone app that has collected data from a person’s fitness-tracker watch. Someone may get timely help for an emotional illness because machine learning allowed a sentiment-sensing application to pick up cues during a conversation. The same principles apply on the population level, where machine learning can help detect clusters or track external health factors.”
Looking forward, Yang concluded, “(p)ersonalization presents itself in countless ways: developing personalized cancer treatments, diagnosing rare diseases, simply allowing caregivers to actually ‘give care,’ and ways we can only begin to imagine today.”
Photo by mikkelwilliams/Getty Images