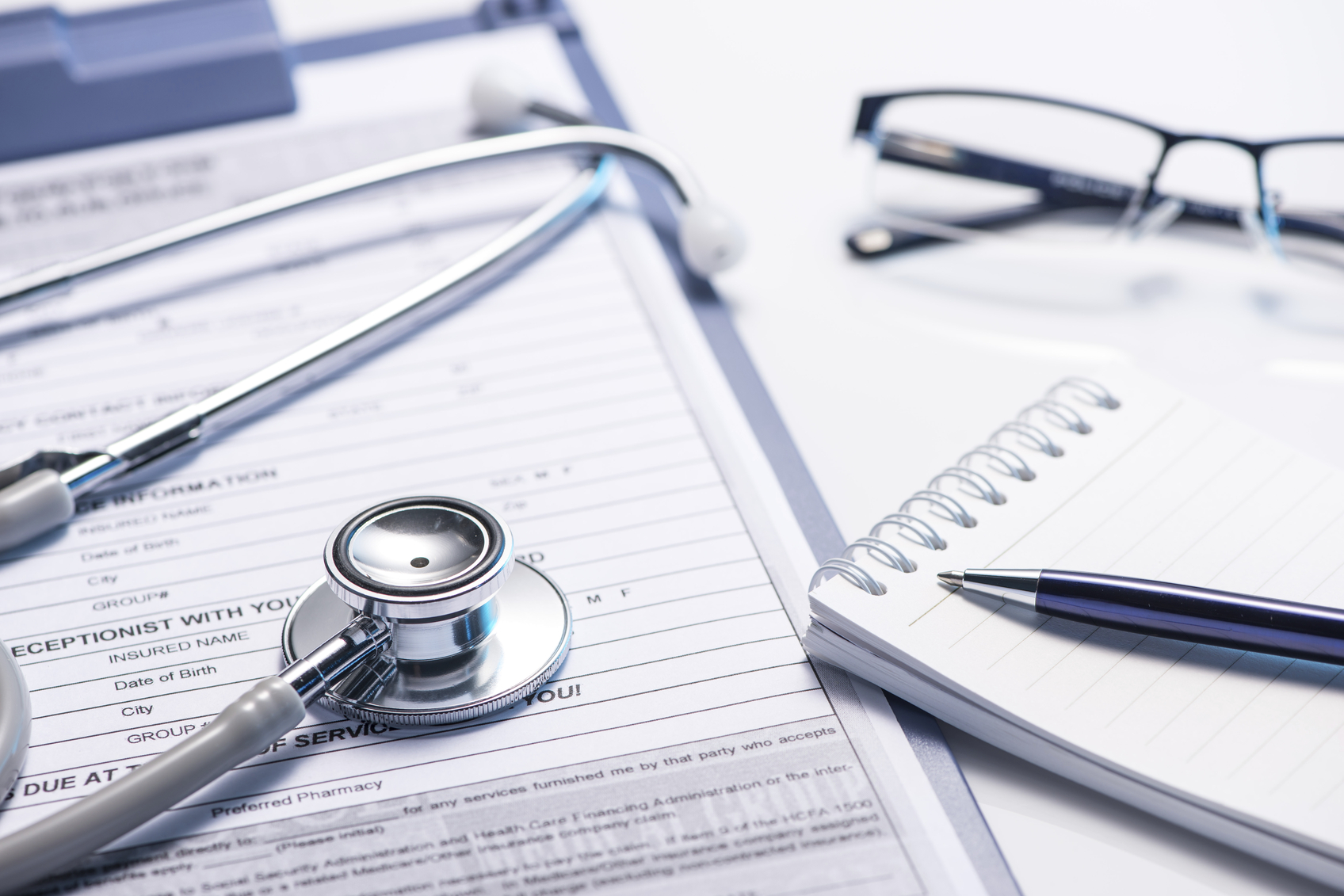
There’s an abundance of discussion underway currently about the importance of ethical uses of IT, but some stakeholders argue those discussions need to take place earlier in the development process.
For example, Kevin G. Ross, CEO of Auckland, New Zealand-based Precision Driven Health, argued recently in an interview with HealthcareITNews, “As with any tool that is introduced into patient care, machine learning should be evaluated on the benefits and risks to patient and provider. Ethics describes our value system and machine learning means using computational power to build models and make decisions on our behalf. As gatekeepers for patient care decisions, clinicians will not adopt or recommend machine learning unless it aligns with their values and builds upon their trusted foundation."
Ross will be sharing his ideas in much greater detail on August 10 at HIMSS21 in Las Vegas in his session titled Ethical Machine Learning.
In his view, what makes machine learning challenging is the evolutionary nature of algorithms. A new device or drug can usually be evaluated in a relatively well-established path of clinical trials, but a machine learning algorithm may perform quite differently today from yesterday, while also producing quite different results for different people and contexts.
"When we allow machine learning to contribute to decision-making, we are introducing an element of real-time research that doesn't easily replicate the rigor of our traditional research evaluation studies," he explained. "Therefore we must, from the very conceptual design stage, think about the ethical implications of our new technologies."
Ethical questions, in other words, should be integrated into the design and implementation of machine learning models to ensure models are developed to maximize benefit and avoid potential harm. To wit, how does one protect privacy, account for inherent bias, ensure that the right people benefit and explain complex models?
Currently, Ross suggest, it’s too easy to get lost in the science of building great models and completely miss both opportunities and risks that the models create.
"Two of the most important processes are a traditional peer review, where someone who understands the data science looks closely at the model and its assumptions, and a risk assessment with the help of a nontechnical person," he said. "Asking a consumer, clinician or planner how they expect a model to be used may identify completely unexpected uses. Documenting what you believe could be the consequence of releasing a model – then monitoring what happens when you do – is an important practice that allows each model to continuously improve through its lifecycle. . . Our techniques are designed and measured on their ability to replicate the past. But what if the past isn't ideal?”
Photo by Makidotvn/Getty Images