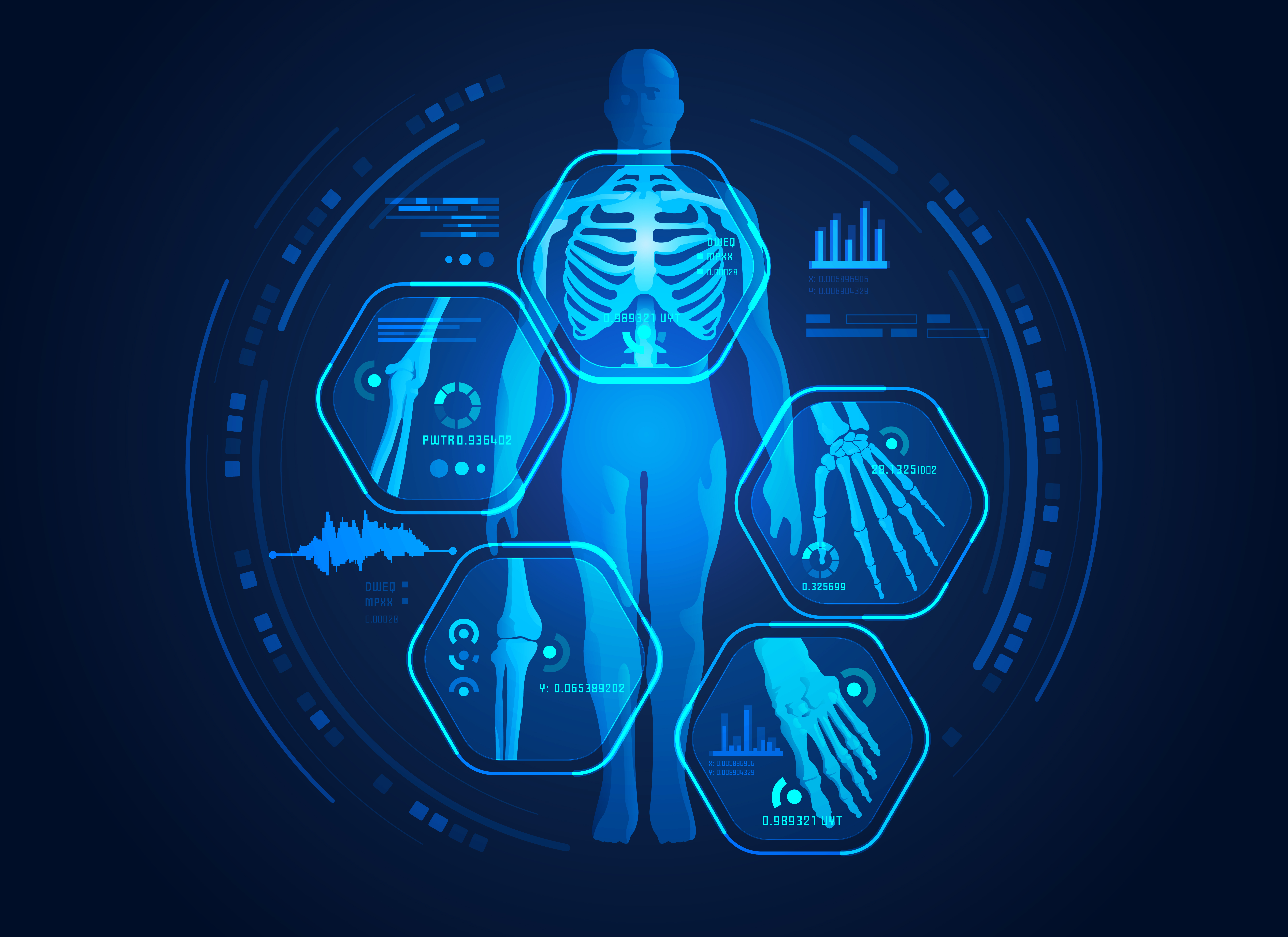
Google has announced that a deep learning tool built by their researchers has detected lung cancer as well as or better than human radiologists, thus demonstrating the potential for AI to boost chances of survival for patients at risk.
The announcement comes on the heels of a study published in Nature Medicine in which researchers said that lung cancer caused an estimated 160,000 deaths in 2018, making it the most common cause of cancer death in the US. Part of the problem, researchers note, is that not enough patients are getting screened in an attempt to catch the disease in its earliest stages.
“Despite the value of lung cancer screenings, only 2-4 percent of eligible patients in the US are screened today,” Shravya Shetty, MS, lead author on the Google study wrote in a blog post. “This work demonstrates the potential for AI to increase both accuracy and consistency, which could help accelerate adoption of lung cancer screening worldwide.”
Currently, lung cancer screenings that use low-dose tomography have been shown to reduce mortality by 20-43 percent, but there are still challenges that can lead to unclear diagnoses, subsequent unnecessary procedures, and high costs. In addition, radiologists often have to look through dozens of 2D images within a single CT scan, and cancer can be hard to spot.
For the Google study, the researchers developed a model using 45,856 de-identified chest CT screening cases from NIH’s National Lung Screening Trial study and Northwestern University, then compared the model’s performance to that of six board-certified radiologists.
When using a single CT scan for diagnosis, the model performed on par or better than human radiologists. The algorithm demonstrated a state-of-the-art performance, at 94.4 percent AUC. The model also reduced false positives by 11 percent and false negatives by five percent.
In addition to determining a patient’s overall lung malignancy, the model can detect subtle malignant tissue in the lungs. The deep learning algorithm can also factor in information from previous scans, which is helpful because the growth rate of suspicious tissue can be indicative of malignancy.
Although the research seems to point directly to the strength and promise of deep learning, the group noted that more investigation will be necessary before the technology can be used in real-world settings.
“These initial results are encouraging, but further studies will assess the impact and utility in clinical practice,” Shetty concluded. “We’re collaborating with Google Cloud Healthcare and Life Sciences team to serve this model through the Cloud Healthcare API and are in early conversations with partners around the world to continue additional clinical validation research and deployment.”