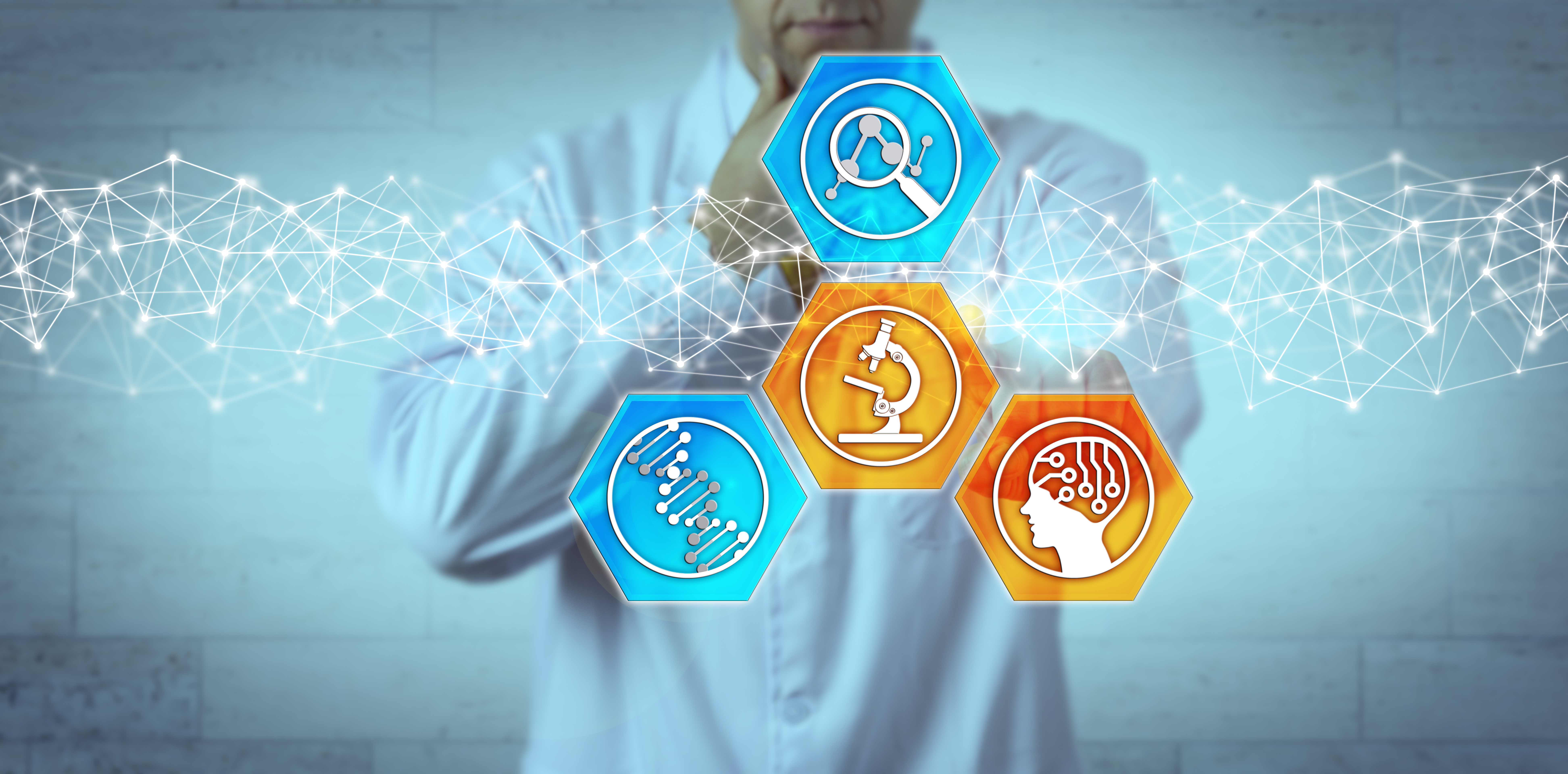
“It is important to emphasize that AI and machine learning will not solve all of healthcare’s problems. That will require an artful blend of artificial and human intelligence, as well as a healthy dose of emotional intelligence.”
So write John Halamka, MD, the newly appointed president of the Mayo Clinic Platform, and healthcare writer Paul Cerrato in a blog post describing their new book, Reinventing Clinical Decision Support.
The focus of the book, they say, is to explore at length the topics of AI and machine learning “with plain clinical English explanations of convolutional neural networks, back propagation, and digital image analysis.”
They provide numerous examples of how these tools are being employed, “including the landmark Google study that demonstrated the value of deep learning in diagnosing diabetic retinopathy. Machine learning–enabled neural networks are also helping to detect melanoma, breast cancer, cancer metastasis, and colorectal cancer, and to manage severe sepsis. AI is even helping to address the opioid epidemic by reducing the number of pills being prescribed postoperatively. Each of these topics includes detailed references to the peer-reviewed medical literature.”
At the same time, they note that that despite the enthusiasm surrounding AI in the healthcare community, it’s important to remember the “criticisms, obstacles, and limitations of these new tools.”
Among the criticisms discussed is ‘the relative lack of hard scientific evidence supporting some of the latest algorithms and the ‘explainability’ dilemma. Most machine learning systems are based on advanced statistics and mind-bending mathematical equations, which have made many clinicians skeptical about their worth.”
According to reviews of the book, the two authors also make the case that “any attempt to reinvent (Clinical Decision Support) also needs to tackle the outdated paradigm that still serves as the underpinning for most patient care”—namely that the “reductionistic mindset (that) insists that most diseases have a single cause.”
In addition, Halamka and Cerrato charge that the “current medical model relies too heavily on a population-based approach to medicine” and that this “one-size-fits-all model is being replaced by a precision medicine approach that takes into account a long list of risk factors.”
Halamka and Cerrato, who also previously co-authored a 2017 book entitled Realizing the Promise of Precision Medicine: The Role of Patient Data, Mobile Technology and Consumer, note that their “enthusiastic take on digital innovation should not give readers the impression that AI will ever replace a competent physician.” Still, they add, there is “little doubt that a competent physician who uses all the tools that AI has to offer will soon replace the competent physician who ignores these tools.”