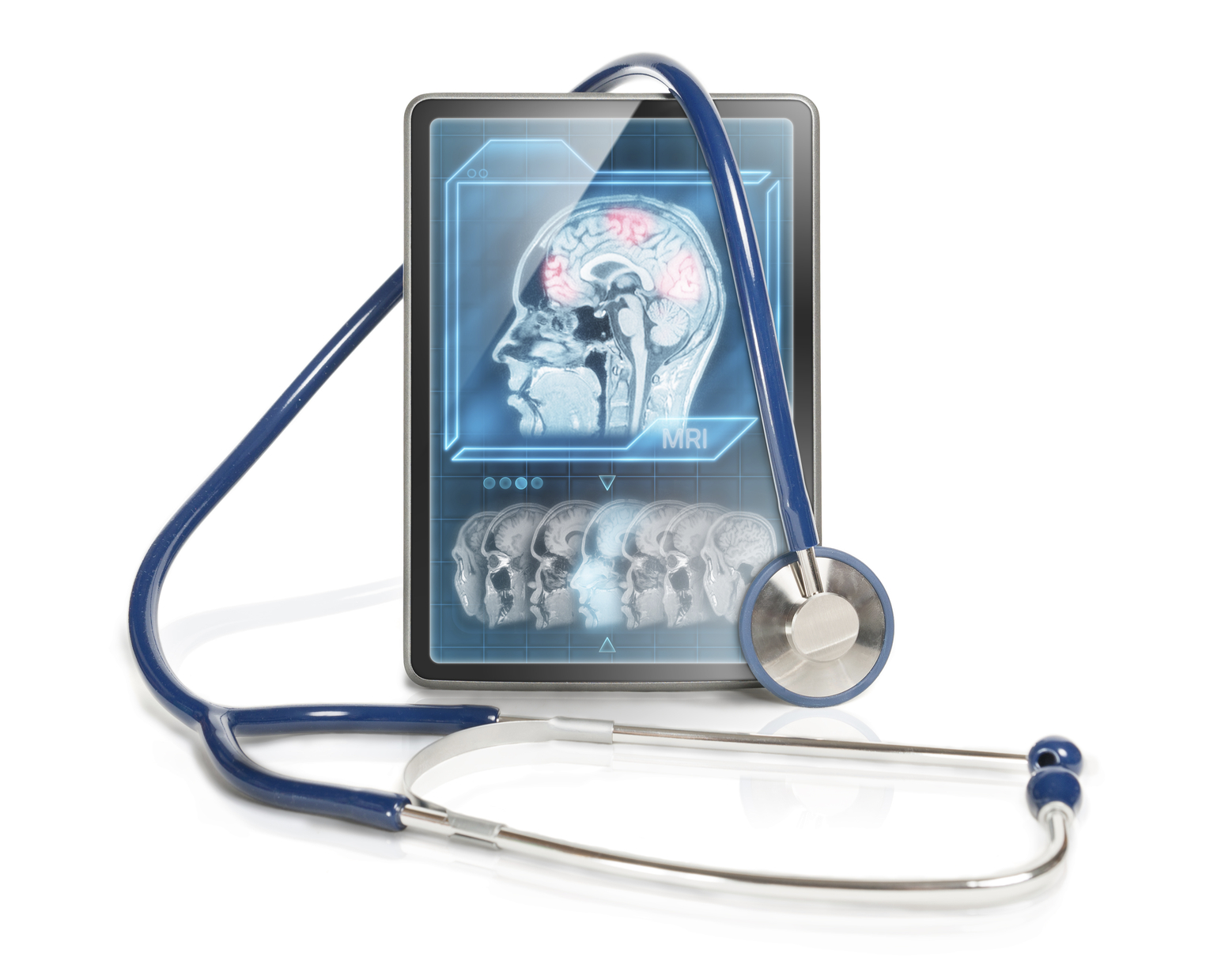
Can AI target lung cancer tumors for radiation therapy treatments as accurately an expert radiation oncologist?
That question was at the heart of a recent crowdsourcing innovation challenge, and answer determined by researchers is that not only can new artificial intelligence algorithms target lung cancer tumors just as well as an expert radiation oncologist, but they can do it 75% to 96% faster.
For the study, researchers from the Dana-Farber Cancer Institute, Brigham and Women’s Hospital, Harvard Catalyst, Harvard Business School and the Laboratory for Innovation Science at Harvard collaborated with network and crowdsourcing platform Topcoder to run the 10-week prize-based contest calling on data scientists to develop AI-based solutions to address the task of tumor segmentation and replicate the accuracy of an expert radiation oncologist.
According to the study’s report, published recently at JAMA Network, the contest was launched as part of an effort to help address the global shortage of expert radiation oncologists.
As for the results, the AI algorithms that were developed performed the tumor segmentation tasks at rates between 15 seconds per scan to two minutes per scan compared to radiation oncologists performing manual segmentation at an average of eight minutes per scan. This opens up the potential for these AI solutions to improve cancer care globally by transferring the skills of expert clinicians to under-resourced health care settings.
According to the report, while AI has been successfully applied to diagnostic subspecialties of medicine, such as pathology and radiology, “applying AI techniques to therapeutic processes in medicine has not been equally well explored because of a lack of large data sets that are well curated by medical experts, and the need for AI techniques capable of adjusting to alterations in practice patterns or risk tolerance and style of individual treating physicians for specific diseases.”
In particular, segmenting tumors, essentially outlining tumors to effectively hit them with radiation without damaging nearby organs, is a time-consuming and resource-intensive task requiring substantial subspecialty training, a problem compounded by the global shortage of experts.
The ultimate goal is to take these AI algorithms and deploy them in the clinical setting.
“This kind of tool also opens the door to innovative applications in the areas of clinical training, quality assurance, and multi-institutional research trials," said Eva Guinan, M.D., professor of radiation oncology at Dana-Farber Cancer Institute and Harvard Medical School and the report’s senior author.