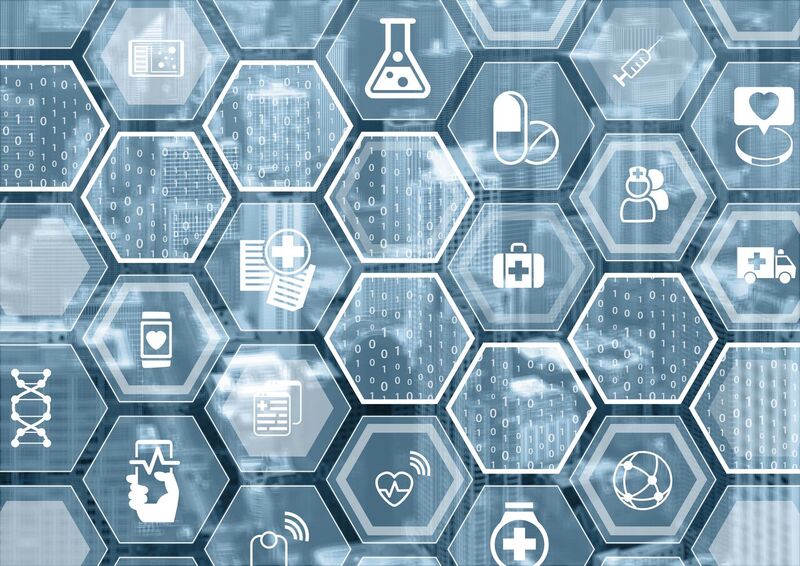
As the leading cause of cancer deaths year in and out, it’s no surprise lung cancer is a constant focus of researchers developing new diagnostic and therapeutic technologies.
To that end, researchers from Radboud University Medical Center in Nijmegen, the Netherlands, along with a team of collaborators, have developed an algorithm for lung nodule assessment using deep learning.
For their study, the report on which was published in the journal Radiology in a paper titled, “Deep Learning for Malignancy Risk Estimation of Pulmonary Nodules Detected at Low-Dose Screening CT, the researchers trained the algorithm on CT images of more than 16,000 nodules, including 1,249 malignancies, from the National Lung Screening Trial, then validated the algorithm on three large sets of imaging data of nodules from the Danish Lung Cancer Screening Trial.
According to a statement accompanying the report, “The deep learning algorithm showed excellent performance, comparable to thoracic radiologists, for malignancy risk estimation of pulmonary nodules detected at screening CT.”
Low-dose chest CT is used to screen people at a high risk of lung cancer, and has been shown to reduce lung cancer mortality by detecting cancers early.
“The algorithm may aid radiologists in accurately estimating the malignancy risk of pulmonary nodules,” added the study’s first author, Kiran Vaidhya Venkadesh, a PhD candidate with the Diagnostic Image Analysis Group at Radboud University Medical Center. “This may help in optimizing follow-up recommendations for lung cancer screening participants.”
The algorithm may also bring several additional benefits, the researchers said.
"As it does not require manual interpretation of nodule imaging characteristics, the proposed algorithm may reduce the substantial interobserver variability in CT interpretation," said senior author Colin Jacobs, Ph.D., assistant professor in the Department of Medical Imaging at Radboud University Medical Center in Nijmegen. "This may lead to fewer unnecessary diagnostic interventions, lower radiologists' workload and reduce costs of lung cancer screening.”
According to the World Health Organization, there were an estimated 1.8 million deaths in 2020 from lung cancer, which accounts for more annual cancer deaths than colon, breast, and prostate cancer combined.
In addition to the recent algorithm, Dr. Jacobs and colleagues have developed other algorithms to reliably extract imaging features from the chest CT related to chronic obstructive pulmonary diseases and cardiovascular diseases. They will be investigating how to effectively integrate these imaging features into the current algorithm.
They also plan to continue improving the latest algorithm by incorporating clinical parameters such as age, sex and smoking history.
Photo by a-image/Getty Images