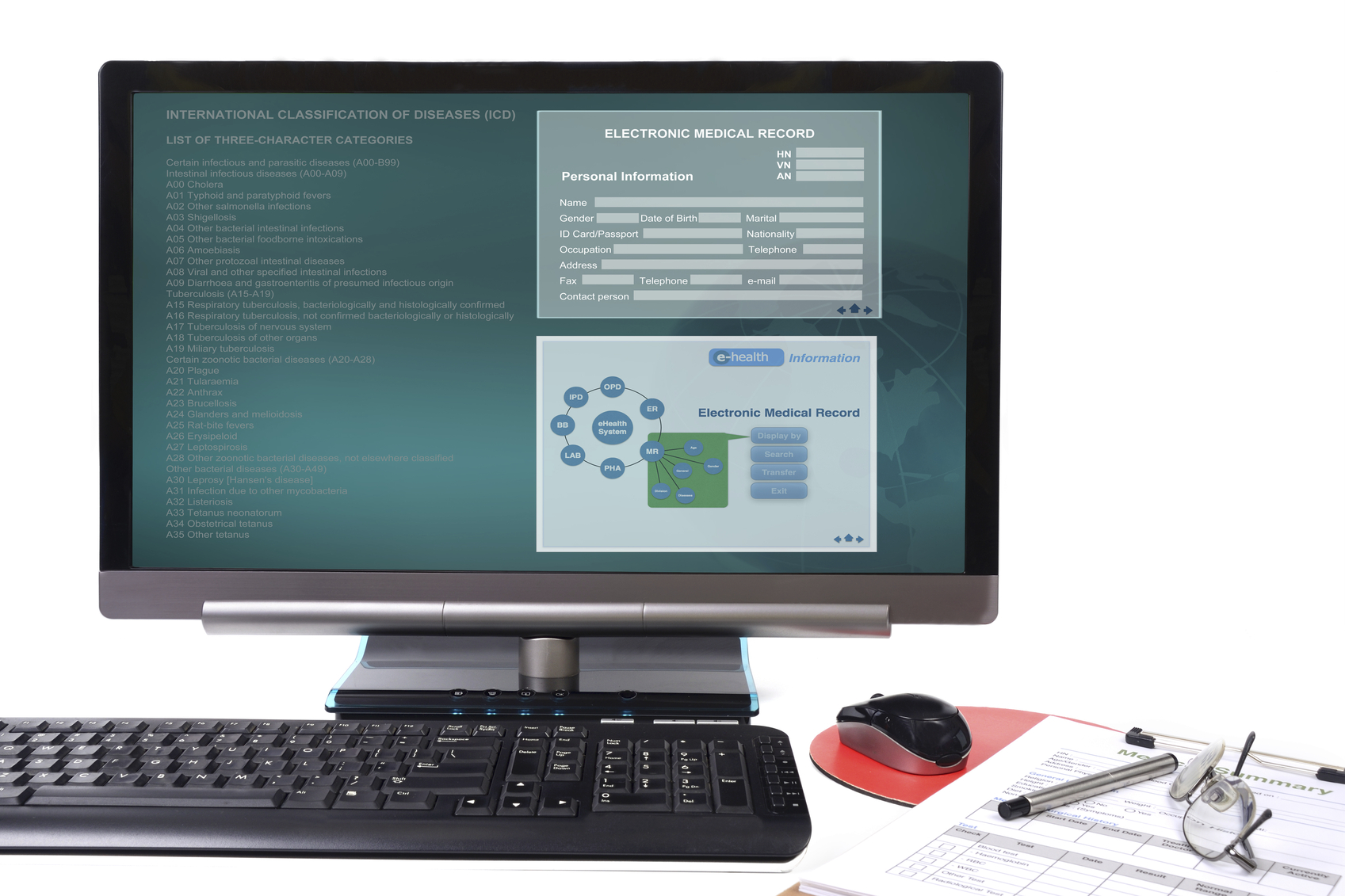
Electronic Health Records (EHRs) are used to capture copious amounts of patient data, and AI algorithms need data on which to learn specific analytic and diagnostic techniques. Should be a match made in heaven, right?
Unfortunately, not so much, says a team of Canadian and U.S. researchers.
Writing recently in The Lancet, the team, from the University of Toronto, Johns Hopkins University and MIT, among other places, argue that while EHRs are an often-tempting repository of data for AI developers, the fact is that “(a)lmost exclusively, EHR data are documented without consideration of the development of algorithms. Data can be collected from a wide range of sources, from high-frequency signals sampled every 0·001 seconds, to vital signs noted hourly, imaging or laboratory tests recorded when needed, notes written at care transitions, and static demographic data. Longitudinal clinical data, such as hospital records for all patients with a particular disease, are similarly heterogenous in data type, time scale, sampling rate, and reason for collection. Each data type is associated with its own challenges.”
Among the challenges they proceed to describe are variation in signal or frequency for many automatically gathered data such as EKG results, irregular ordering patterns for imaging data, vital signs and lab tests, and often sketchy thoroughness or accuracy for narrative clinical notes.
Digging deeper into three specific challenges, the writers note, first, “clinical data tend to be messy, incomplete, and potentially biased. Imputation, sparse encoding, or matrix factorization methods can be used to address incomplete or missing data features, but they must be used with caution because the correct method depends on which data are missing. Robust inference also depends on large, representative datasets. However, gaining access to a sufficient amount of data in a health-care setting can be challenging because of patient privacy restrictions.”
Second, they say, “if outcomes are predicted on the basis of measurements, problems can arise when the measurements change considerably. For example, a model trained on data from an urban hospital might not be able to predict outcomes in a rural setting. Measurements can change over time as patient populations change or care policies evolve. Data might unintentionally be confounded by measurement drift as equipment ages or changes, which can be adjusted for if the drift effect is identified. Unsupervised learning methods or causal inference approaches could potentially be used to detect shifts in the underlying population.”
Finally, the very identification, or labelling, of diseases can vary from provider to provider, thus leading to potential inconsistencies in the data on which AI algorithms are being “taught.”
In the long run, the team says, the parameters of any data used for AI “training” must be explicitly defined when building each algorithm, and clinicians and AI researchers should “work collaboratively to pair clinical challenges with novel technical solutions. Engaging in close partnerships will create meaningful algorithms, foster community, and form culture.”