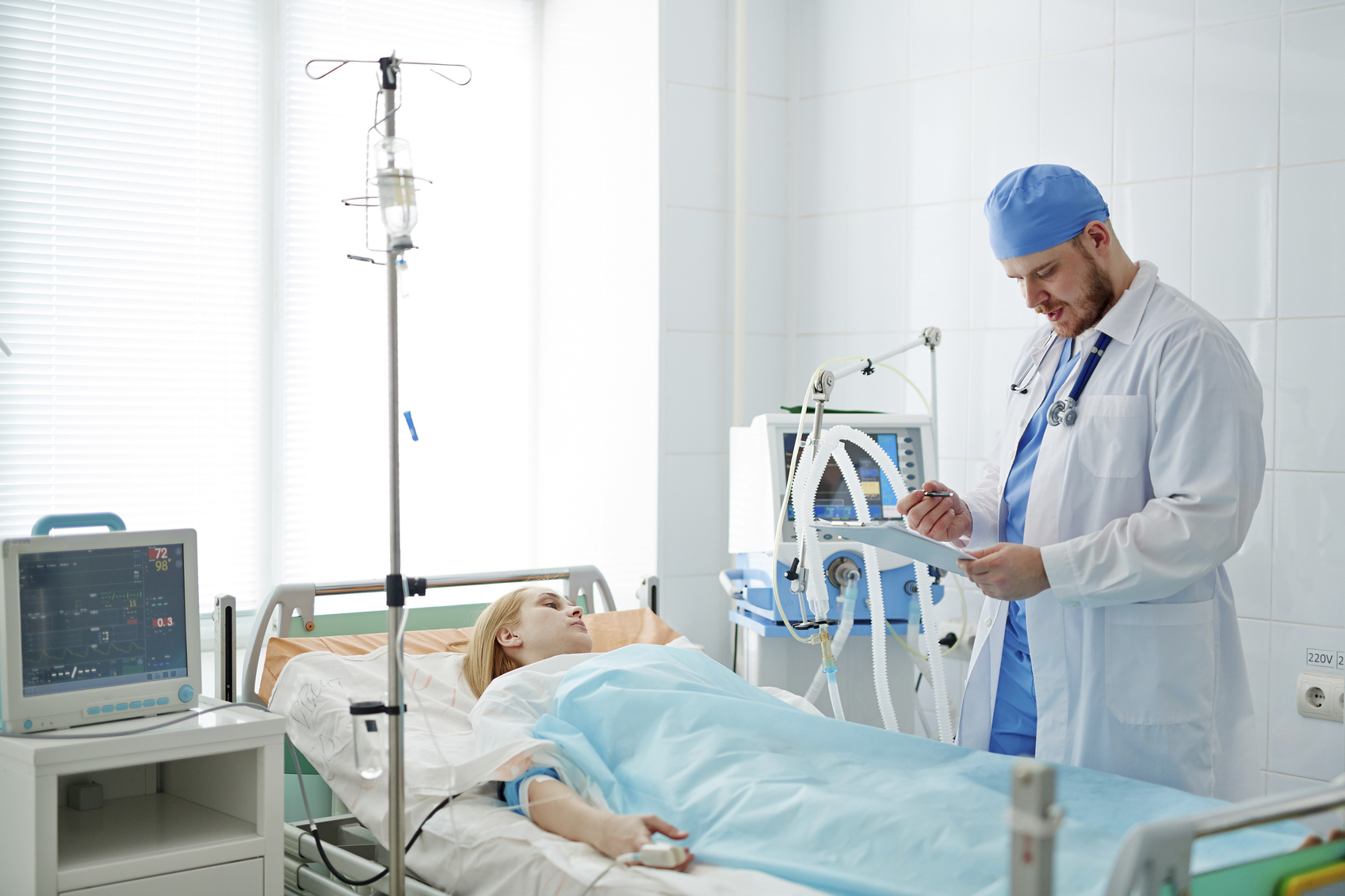
Isolating and identifying a symptom is generally the first step toward determining how best to treat whatever is causing it, so for sufferers of chronic pain a recent study by University of Pittsburgh researchers may be a forerunner of some greatly desired relief.
According to a report published recently in the open-access journal PLOS ONE, pain distribution as reported on a body map can be used to assign patients to distinct subgroups that are associated with differences in pain intensity, pain quality and pain impact, thus potentially identifying a path toward better, more personalized, medicine.
“The experience of pain is complex and personal, making it difficult to communicate and quantify,” the team noted at the beginning of their report. “Despite these measurement challenges, parsing the experience into distinct constructs has afforded considerable progress in grouping chronic pain syndromes, defining chronic pain as a disease, and tailoring pain treatments.”
For the study, researchers examined data from 21,658 patients at the University of Pittsburgh’s seven pain management clinics between 2016 and 2019. Every patient completed a pain body map, selecting areas of pain on two side-by-side drawings of the front and back of a body. In total, the map identified 74 possible regions of pain.
Moreover, other information on patients’ pain, health, and outcomes were collected from relevant EHRs.
According to the report, “(t)he goals of the current study were (1) to identify discrete patterns of patient-reported pain distribution in chronic pain patients, and (2) to determine the relationship(s) of these patterns with pain intensity, impact, and clinical outcome. . . Rather than relying on prior diagnostic classifications, our approach was to utilize hierarchical clustering as a way to identify distinct groups of patients by similarities in body map selection alone.”
Data from all patients revealed 9 distinct groupings of pain distribution. Demographic and medical characteristics, pain intensity, pain impact, and neuropathic pain quality all varied significantly across cluster subgroups.
For instance, explained a press release accompanying the report, “the pain intensity of the ‘Neck and Shoulder’ group was less than that of ‘Lower Back Pain below knee’ and ‘Neck, Shoulder and Lower Back Pain,’ while the group with the highest pain intensity consisted of patients with widespread heavy pain, also associated with low physical function, high anxiety and depression and high sleep disturbance.”
In a group of 7,138 patients who completed three-month follow-up questionnaires, subgroups predicted the likelihood of improvement in pain and physical function. Individuals in the “Abdominal Pain” group were most improved, with 49 percent self-reporting significant improvements.
“Using an algorithmic approach, we found that how a patient reports the bodily distribution of their chronic pain affects nearly all aspects of the pain experience, including what happens three months later,” the researchers concluded. “This emphasizes that chronic pain is a disease process and suggests that this facet of the chronic pain phenotype will be important for future developments in diagnosis and personalized pain management.”
Photo by shironosov/Getty Images