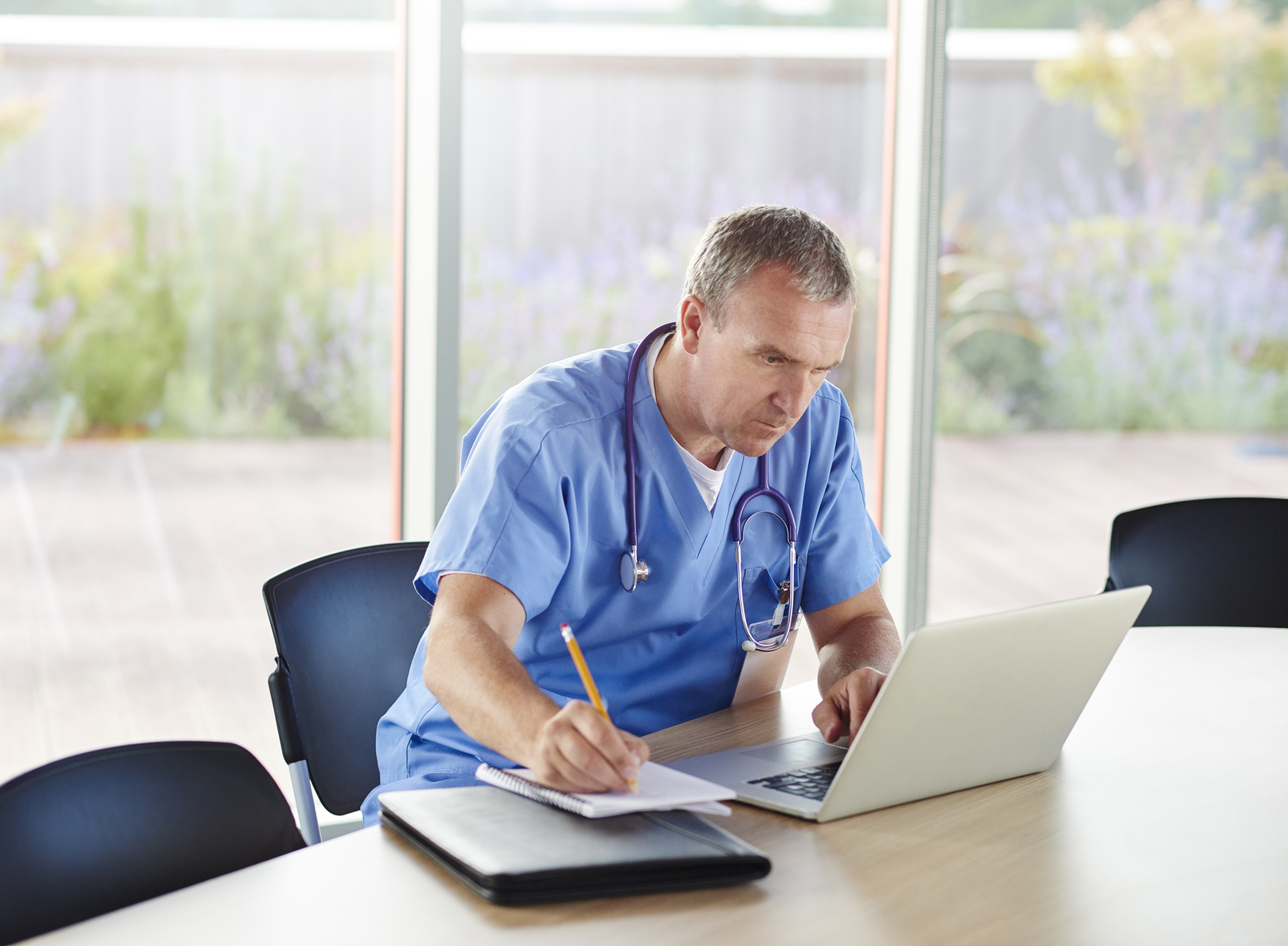
In what is being touted as the first use of its kind of AI network analysis, a new study, published by Nature Scientific Reports, describes how a team of scientists from the University of Surrey and the University of California examined the structure and relationships between 38 common symptoms reported by over 1300 cancer patients receiving chemotherapy.
According to the report, “(o)ncology patients undergoing cancer treatment experience an average of fifteen unrelieved symptoms that are highly variable in both their severity and distress. In order to advance symptom management science and gain a better understanding of oncology patients’ symptom experiences, research has focused on the evaluation of symptom clusters using techniques such as exploratory factor analysis or cluster analysis. One of the underlying assumptions of this research is that symptoms that cluster together may share underlying mechanisms that are potential targets for therapeutic interventions. While progress is being made in symptom clusters research, one of the major gaps in knowledge using standard statistical approaches is that the nature of the relationships among individual symptoms and symptom clusters have not been evaluated.”
The ability for AI to "learn" from the data provides the opportunity for improved accuracy based on feedback responses, stakeholders note, feedback that includes many backend database sources, input from practitioners, doctors, and research institutions.
In a statement, the University of California’s Christine Miaskowski noted, "This fresh approach will allow us to develop and test novel and more targeted interventions to decrease symptom burden in cancer patients undergoing chemotherapy.”
In healthcare, experts explain, AI systems are always working in real time, constantly updating data and thus increasing accuracy and relevance. For purposes of studies such as this, then, assembled data is a compilation of different medical notes, electronic recordings from medical devices, laboratory images, physical examinations and various demographics.
Moreover, machine learning techniques can use analytical algorithms in order to pull out specific patient traits, which include all the information that would be collected in a patient visit with a practitioner.
According to the report’s authors, “we believe that NA has the potential to improve our understanding of the oncology patients’ symptom experience so that individualized and targeted interventions can be prescribed to reduce each patient’s symptom burden.”